Learn How to Train ChatGPT Quickly and Easily
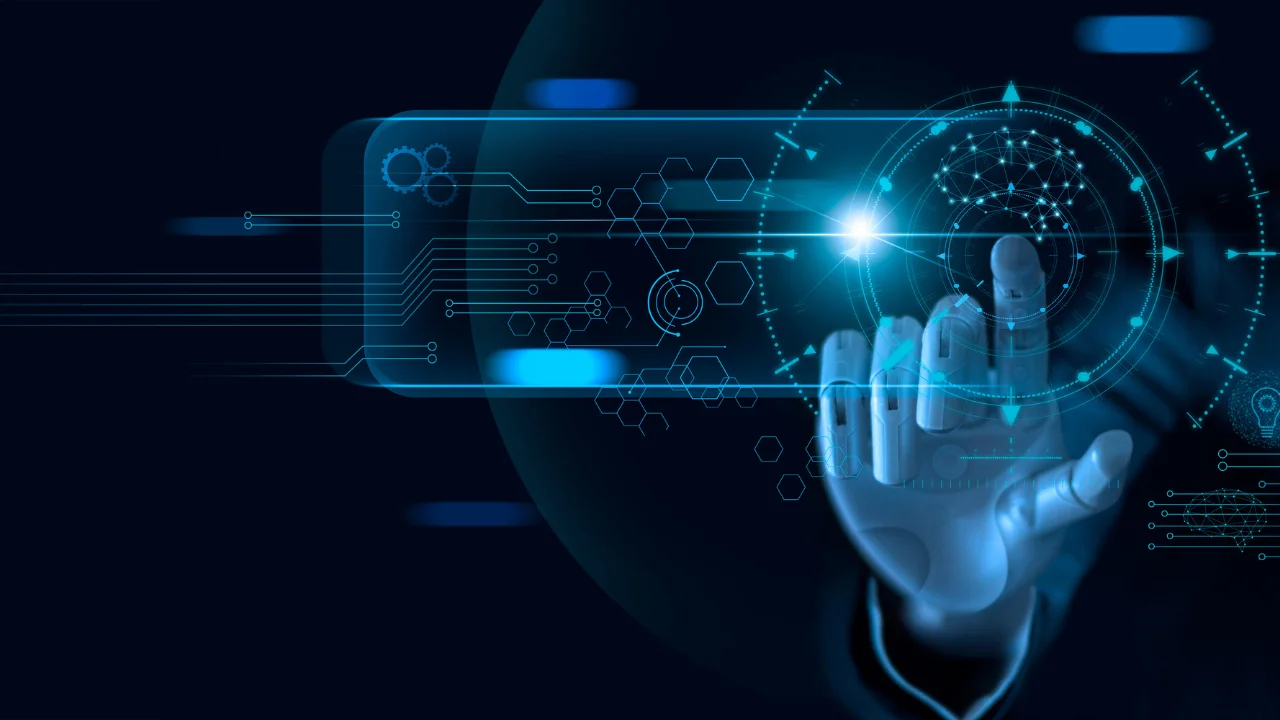
Are you looking to enhance ChatGPT's capabilities? Look no further! In this blog, Musketeers Tech will provide a step-by-step guide on how to train ChatGPT quickly and easily. Whether you are a developer or an AI enthusiast, our methods will help you make the most of this powerful language model.
Training ChatGPT can be a complex task, but with our easy-to-follow instructions, you can navigate the process seamlessly. We'll cover everything from preparing the training data to fine-tuning the model, ensuring you have a robust and effective conversational AI system.
Leveraging the power of ChatGPT, you can create chatbots, virtual assistants, and more. Its ability to understand and generate human-like text opens up a world of possibilities for your applications. So, if you're ready to dive into the world of training ChatGPT, let's get started.
The Importance of Training ChatGPT
Training a language model like ChatGPT is crucial to ensure it performs well and generates high-quality responses. While ChatGPT comes pre-trained on a vast amount of internet text, fine-tuning it with specific data tailored to your use case can significantly improve its performance.
When you train ChatGPT, you can shape its behaviour and responses. By exposing the model to relevant and high-quality training data, you can guide it to generate more accurate and contextually appropriate responses. This is especially important for chat-based applications, where users expect conversational interactions that feel natural and human-like.
With proper training, it may produce consistent outputs. By investing time and effort into training your model, you can ensure that it aligns with your desired behaviour and meets your users' needs.
Training ChatGPT is not a one-time task but an ongoing process. As you collect more user feedback and iterate on your model, you can continually improve its performance and address potential limitations or biases.
In the next section, we will dive into the training process for ChatGPT and explore the steps involved in creating a well-trained conversational AI system.
Understanding the Training Process for ChatGPT
The training process for ChatGPT involves several vital steps to ensure that the model learns and generalizes from the provided data. Let's take a closer look at each of these steps.
Data Collection: The first step in training ChatGPT is to gather relevant and diverse training data. This data will serve as the foundation for teaching the model how to respond accurately to user inputs. You can collect data from various sources, such as chat logs, customer support interactions, or even by creating conversational datasets.
Data Cleaning and Formatting: Once you have collected the training data, it's essential to clean and format it appropriately. This involves removing irrelevant or noisy data, correcting spelling or grammatical errors, and ensuring consistent formatting. Clean and well-structured data will help the model learn more effectively and produce better responses.
Data Tokenization: Before training, the text data needs to be tokenized, which involves splitting the input text into smaller units called tokens. Tokenization helps the model process and understand the text more efficiently. For example, the sentence "I love ChatGPT!" could be tokenized into ["I", "love", "Chat", "G", "PT", "!"].
Model Training: Once the data is prepared, it's time to train the ChatGPT model. This process involves feeding the tokenized data into the model and updating its parameters through backpropagation. During training, the model learns to predict the next token in a sequence based on the provided context, allowing it to generate coherent and contextually relevant responses.
Evaluation and Fine-tuning: After training, it's crucial to evaluate the performance of the trained model. This can be done using a separate validation dataset or collecting user feedback. By analyzing the model's responses and identifying areas for improvement, you can fine-tune the model to enhance its accuracy and generate more satisfactory outputs.
Now that we have a better understanding of the training process for ChatGPT, let's explore how to prepare your training data to ensure practical training in the next section.
Preparing Your Training Data for ChatGPT
The quality and relevance of your training data play a vital role in the performance of the ChatGPT model. To maximize the virtue of your training, consider the following steps when preparing your training data.
Data Filtering: Before starting the training process, filtering out irrelevant or low-quality data is essential. Removing noisy or biased inputs can significantly improve the model's performance and prevent it from generating inaccurate or inappropriate responses. Additionally, be mindful of any sensitive or personally identifiable information in the data and ensure it is adequately anonymized or removed.
Diverse and Balanced Data: To train a robust and versatile ChatGPT model, aim to include a diverse range of examples in your training data. This helps the model handle conversational scenarios and adapt to user inputs. Additionally, ensure a balance between positive and negative examples to prevent the model from becoming overly biased or one-sided in its responses.
Contextual Data: Including relevant context in your training data is crucial for training a conversational AI system. Context helps the model understand the flow of the conversation and generate appropriate and coherent responses. When preparing your data, include previous user inputs and system responses to provide the necessary context for the model to learn from.
Data Augmentation: If you have limited training data, consider augmenting it by generating additional examples. This can be achieved by paraphrasing existing conversations, introducing sentence structure or word choice variations, or using dialogue generation models to create new discussions. Data augmentation helps increase the diversity of your training data and improves the model's ability to handle different input patterns.
Following these steps, you can ensure that your training data is clean, relevant, and diverse, setting the foundation for practical ChatGPT training. The following section will discuss strategies for choosing suitable prompts to enhance the training process further.
Choosing the Right Prompts for Effective Training
The choice of prompts used during training can significantly impact ChatGPT's performance and behaviour. Prompts serve as the initial instructions or context provided to the model, guiding its responses. Here are some strategies to consider when selecting prompts for training.
Clear and Specific Prompts: Ensure that your prompts provide clear and specific instructions to the model. Ambiguous or vague prompts may result in inconsistent responses. You can guide the model to generate more accurate and helpful outputs by being explicit in your prompts.
Varied Prompt Styles: To improve the model's ability to handle different conversational styles, consider using a variety of prompt styles during training. For example, you can include casual prompts, formal prompts, prompts with specific personas, or prompts that require the model to answer factual questions. This helps the model generalize better and adapt to various user preferences.
Prompt Length: Experiment with different prompt lengths to find the optimal balance. Short prompts may need more context for the model to generate meaningful responses, while excessively long prompts may overwhelm the model and lead to less coherent outputs. Fine-tune the prompt length based on your specific use case and the desired response complexity.
Interactive Prompts: Incorporating interactive prompts during training can simulate a conversational setting and improve the model's ability to engage in dynamic interactions. By including back-and-forth exchanges in your training data, you can train the model to handle conversation flows, interruptions, and follow-up questions.
Choosing the proper prompts is an iterative process. Experiment with different prompt strategies and evaluate the model's responses to refine your training approach. In the next section, we will discuss strategies for improving the quality of ChatGPT responses.
Strategies for Improving the Quality of ChatGPT Responses
While training ChatGPT can enhance its performance, there are additional strategies you can employ to improve the quality of its responses further. These strategies focus on post-processing techniques and fine-tuning the model's behaviour.
Response Filtering: Implementing a response filtering mechanism can help prevent the model from generating inappropriate or harmful content. By defining a set of guidelines or rules for acceptable responses, you can filter out any outputs that violate these guidelines. This ensures that the generated responses align with your desired behaviour and remain safe for users.
Contextual Re-ranking: After the model generates a response, you can use context-based re-ranking techniques to select the most suitable output. This involves comparing the generated response with the context and selecting the most relevant or coherent response. Contextual re-ranking can help refine the model's outputs and ensure they align with the conversation flow.
System-Level Control: Incorporating system-level control mechanisms allows you to influence ChatGPT's behaviour. You can add instructions or constraints to guide the model's responses, such as specifying a desired level of formality, tone, or domain expertise. System-level control helps fine-tune the model to meet specific requirements and ensures consistent interaction behaviour.
Iterative Feedback Loop: Establishing an iterative feedback loop with real users is crucial for improving the quality of ChatGPT responses. Collect user feedback on the generated outputs and continuously iterate on your model. You can address any limitations or biases by analyzing user interactions, refining the training data and prompt strategies, and enhancing ChatGPT's overall performance.
By applying these strategies, you can refine and improve the quality of ChatGPT responses, making them more reliable and suitable for your specific use case. In the next section, we will explore the process of evaluating and fine-tuning your trained ChatGPT model.
Evaluating and Fine-tuning Your Trained ChatGPT Model
Assessing the performance of your trained ChatGPT model is crucial to ensure its effectiveness and identify areas for improvement. Here are some steps to evaluate and fine-tune your trained model.
Evaluation Metrics: Define appropriate evaluation metrics to assess your model's quality and performance. Metrics such as perplexity, BLEU score, or human evaluation can provide insights into the model's language fluency, coherence, and overall accuracy. Additionally, consider collecting user feedback to gauge user satisfaction and identify areas for improvement.
Validation Dataset: Set aside a separate validation dataset to evaluate the model's performance. This dataset should consist of conversations or input-output pairs not included in the training data. By considering the model on unseen data, you can assess its ability to generalize and produce accurate responses.
Fine-tuning Techniques: If the evaluation reveals areas for improvement, you can fine-tune the model to address these issues. Fine-tuning involves retraining the model using specific techniques such as reinforcement learning, curriculum learning, or adversarial training. Fine-tuning allows you to refine the model's behaviour and performance on specific tasks or domains.
Continuous Improvement: The process of training and fine-tuning ChatGPT is iterative. Collect user feedback on the model's performance in real-world scenarios and use it to improve it further. Consider implementing an efficient feedback loop to continuously update and refine the training data, prompts, or post-processing techniques based on user interactions.
Regular evaluation and fine-tuning are essential to ensure your trained ChatGPT model remains up-to-date, accurate, and aligned with your desired behaviour. The following section will provide some tips for efficient and quick ChatGPT training.
Tips for Efficient and Quick Training of ChatGPT
Training ChatGPT can be time-consuming, but you can employ several tips and techniques to make it more efficient and faster. Consider the following suggestions to streamline your training.
Hardware Acceleration: Use hardware acceleration, such as GPUs or TPUs, to speed up the training process. These specialized hardware devices are designed to perform matrix computations efficiently, which are fundamental to training deep learning models like ChatGPT. Hardware acceleration can significantly reduce the training time and make the process more efficient.
Batch Training: Train your model using mini-batches of data rather than individual examples. Batch training allows the model to process multiple examples simultaneously, leveraging parallelism and reducing the overall training time. Experiment with different batch sizes to find the optimal balance between training speed and memory requirements.
Early Stopping: Implement early stopping techniques during training to prevent overfitting and save training time. Early stopping involves monitoring the model's performance on a validation dataset during training and stopping the training process when the performance degrades. This helps avoid unnecessary training iterations and speeds up the overall process.
Transfer Learning: Leverage transfer learning techniques to accelerate the training process. Instead of training ChatGPT from scratch, start with a pre-trained model and fine-tune it using your specific dataset. Transfer learning allows the model to benefit from the knowledge learned on a large-scale dataset, reducing the training time while still achieving good performance.
By following these tips, you can optimize the training process and reduce the time required to train ChatGPT, making it more efficient and practical for real-world applications. In the next section, we will explore some tools and resources that can aid in training ChatGPT.
Tools and Resources for Training ChatGPT
Training ChatGPT requires access to suitable tools and resources. Fortunately, several libraries, frameworks, and pre-trained models are available to simplify and streamline the training process. Here are some notable tools and resources.
Hugging Face Transformers: The library provides a high-level API for training and fine-tuning transformer models, including ChatGPT. It offers various pre-trained models, tokenizers, and utilities that facilitate the training and deployment of ChatGPT.
OpenAI GPT-3: OpenAI GPT-3 is a state-of-the-art language model for training conversational AI systems. It provides an API that allows developers to interact with and train the model using custom prompts and datasets. GPT-3 offers powerful capabilities for natural language processing and generation.
Datasets: Several datasets are available for training conversational AI systems. The ParlAI framework provides access to various conversational datasets, including dialogue-based tasks and chat.
Conclusion
Training ChatGPT is a complex and multifaceted process that involves data collection, preprocessing, model architecture, training, and fine-tuning. The resulting language model offers powerful capabilities that can be leveraged in numerous practical applications, from customer support and content creation to virtual assistants and educational tools.
While AI language models are associated with challenges and limitations, ongoing research, development, ethical considerations, and bias mitigation efforts will help address these issues. The future of AI language models like ChatGPT is bright, with enhanced capabilities, integration with other technologies, and democratization efforts paving the way for exciting new possibilities.
By understanding how to train and utilize ChatGPT, individuals and organizations can harness the power of AI to drive innovation, improve efficiency, and create value across various domains. As we continue to explore the potential of AI language models, the possibilities are limited only by our imagination.
Are you looking to enhance ChatGPT's capabilities? Look no further! In this blog, Musketeers Tech will provide a step-by-step guide on how to train ChatGPT quickly and easily. Whether you are a developer or an AI enthusiast, our methods will help you make the most of this powerful language model.
Training ChatGPT can be a complex task, but with our easy-to-follow instructions, you can navigate the process seamlessly. We'll cover everything from preparing the training data to fine-tuning the model, ensuring you have a robust and effective conversational AI system.
Leveraging the power of ChatGPT, you can create chatbots, virtual assistants, and more. Its ability to understand and generate human-like text opens up a world of possibilities for your applications. So, if you're ready to dive into the world of training ChatGPT, let's get started.
The Importance of Training ChatGPT
Training a language model like ChatGPT is crucial to ensure it performs well and generates high-quality responses. While ChatGPT comes pre-trained on a vast amount of internet text, fine-tuning it with specific data tailored to your use case can significantly improve its performance.
When you train ChatGPT, you can shape its behaviour and responses. By exposing the model to relevant and high-quality training data, you can guide it to generate more accurate and contextually appropriate responses. This is especially important for chat-based applications, where users expect conversational interactions that feel natural and human-like.
With proper training, it may produce consistent outputs. By investing time and effort into training your model, you can ensure that it aligns with your desired behaviour and meets your users' needs.
Training ChatGPT is not a one-time task but an ongoing process. As you collect more user feedback and iterate on your model, you can continually improve its performance and address potential limitations or biases.
In the next section, we will dive into the training process for ChatGPT and explore the steps involved in creating a well-trained conversational AI system.
Understanding the Training Process for ChatGPT
The training process for ChatGPT involves several vital steps to ensure that the model learns and generalizes from the provided data. Let's take a closer look at each of these steps.
Data Collection: The first step in training ChatGPT is to gather relevant and diverse training data. This data will serve as the foundation for teaching the model how to respond accurately to user inputs. You can collect data from various sources, such as chat logs, customer support interactions, or even by creating conversational datasets.
Data Cleaning and Formatting: Once you have collected the training data, it's essential to clean and format it appropriately. This involves removing irrelevant or noisy data, correcting spelling or grammatical errors, and ensuring consistent formatting. Clean and well-structured data will help the model learn more effectively and produce better responses.
Data Tokenization: Before training, the text data needs to be tokenized, which involves splitting the input text into smaller units called tokens. Tokenization helps the model process and understand the text more efficiently. For example, the sentence "I love ChatGPT!" could be tokenized into ["I", "love", "Chat", "G", "PT", "!"].
Model Training: Once the data is prepared, it's time to train the ChatGPT model. This process involves feeding the tokenized data into the model and updating its parameters through backpropagation. During training, the model learns to predict the next token in a sequence based on the provided context, allowing it to generate coherent and contextually relevant responses.
Evaluation and Fine-tuning: After training, it's crucial to evaluate the performance of the trained model. This can be done using a separate validation dataset or collecting user feedback. By analyzing the model's responses and identifying areas for improvement, you can fine-tune the model to enhance its accuracy and generate more satisfactory outputs.
Now that we have a better understanding of the training process for ChatGPT, let's explore how to prepare your training data to ensure practical training in the next section.
Preparing Your Training Data for ChatGPT
The quality and relevance of your training data play a vital role in the performance of the ChatGPT model. To maximize the virtue of your training, consider the following steps when preparing your training data.
Data Filtering: Before starting the training process, filtering out irrelevant or low-quality data is essential. Removing noisy or biased inputs can significantly improve the model's performance and prevent it from generating inaccurate or inappropriate responses. Additionally, be mindful of any sensitive or personally identifiable information in the data and ensure it is adequately anonymized or removed.
Diverse and Balanced Data: To train a robust and versatile ChatGPT model, aim to include a diverse range of examples in your training data. This helps the model handle conversational scenarios and adapt to user inputs. Additionally, ensure a balance between positive and negative examples to prevent the model from becoming overly biased or one-sided in its responses.
Contextual Data: Including relevant context in your training data is crucial for training a conversational AI system. Context helps the model understand the flow of the conversation and generate appropriate and coherent responses. When preparing your data, include previous user inputs and system responses to provide the necessary context for the model to learn from.
Data Augmentation: If you have limited training data, consider augmenting it by generating additional examples. This can be achieved by paraphrasing existing conversations, introducing sentence structure or word choice variations, or using dialogue generation models to create new discussions. Data augmentation helps increase the diversity of your training data and improves the model's ability to handle different input patterns.
Following these steps, you can ensure that your training data is clean, relevant, and diverse, setting the foundation for practical ChatGPT training. The following section will discuss strategies for choosing suitable prompts to enhance the training process further.
Choosing the Right Prompts for Effective Training
The choice of prompts used during training can significantly impact ChatGPT's performance and behaviour. Prompts serve as the initial instructions or context provided to the model, guiding its responses. Here are some strategies to consider when selecting prompts for training.
Clear and Specific Prompts: Ensure that your prompts provide clear and specific instructions to the model. Ambiguous or vague prompts may result in inconsistent responses. You can guide the model to generate more accurate and helpful outputs by being explicit in your prompts.
Varied Prompt Styles: To improve the model's ability to handle different conversational styles, consider using a variety of prompt styles during training. For example, you can include casual prompts, formal prompts, prompts with specific personas, or prompts that require the model to answer factual questions. This helps the model generalize better and adapt to various user preferences.
Prompt Length: Experiment with different prompt lengths to find the optimal balance. Short prompts may need more context for the model to generate meaningful responses, while excessively long prompts may overwhelm the model and lead to less coherent outputs. Fine-tune the prompt length based on your specific use case and the desired response complexity.
Interactive Prompts: Incorporating interactive prompts during training can simulate a conversational setting and improve the model's ability to engage in dynamic interactions. By including back-and-forth exchanges in your training data, you can train the model to handle conversation flows, interruptions, and follow-up questions.
Choosing the proper prompts is an iterative process. Experiment with different prompt strategies and evaluate the model's responses to refine your training approach. In the next section, we will discuss strategies for improving the quality of ChatGPT responses.
Strategies for Improving the Quality of ChatGPT Responses
While training ChatGPT can enhance its performance, there are additional strategies you can employ to improve the quality of its responses further. These strategies focus on post-processing techniques and fine-tuning the model's behaviour.
Response Filtering: Implementing a response filtering mechanism can help prevent the model from generating inappropriate or harmful content. By defining a set of guidelines or rules for acceptable responses, you can filter out any outputs that violate these guidelines. This ensures that the generated responses align with your desired behaviour and remain safe for users.
Contextual Re-ranking: After the model generates a response, you can use context-based re-ranking techniques to select the most suitable output. This involves comparing the generated response with the context and selecting the most relevant or coherent response. Contextual re-ranking can help refine the model's outputs and ensure they align with the conversation flow.
System-Level Control: Incorporating system-level control mechanisms allows you to influence ChatGPT's behaviour. You can add instructions or constraints to guide the model's responses, such as specifying a desired level of formality, tone, or domain expertise. System-level control helps fine-tune the model to meet specific requirements and ensures consistent interaction behaviour.
Iterative Feedback Loop: Establishing an iterative feedback loop with real users is crucial for improving the quality of ChatGPT responses. Collect user feedback on the generated outputs and continuously iterate on your model. You can address any limitations or biases by analyzing user interactions, refining the training data and prompt strategies, and enhancing ChatGPT's overall performance.
By applying these strategies, you can refine and improve the quality of ChatGPT responses, making them more reliable and suitable for your specific use case. In the next section, we will explore the process of evaluating and fine-tuning your trained ChatGPT model.
Evaluating and Fine-tuning Your Trained ChatGPT Model
Assessing the performance of your trained ChatGPT model is crucial to ensure its effectiveness and identify areas for improvement. Here are some steps to evaluate and fine-tune your trained model.
Evaluation Metrics: Define appropriate evaluation metrics to assess your model's quality and performance. Metrics such as perplexity, BLEU score, or human evaluation can provide insights into the model's language fluency, coherence, and overall accuracy. Additionally, consider collecting user feedback to gauge user satisfaction and identify areas for improvement.
Validation Dataset: Set aside a separate validation dataset to evaluate the model's performance. This dataset should consist of conversations or input-output pairs not included in the training data. By considering the model on unseen data, you can assess its ability to generalize and produce accurate responses.
Fine-tuning Techniques: If the evaluation reveals areas for improvement, you can fine-tune the model to address these issues. Fine-tuning involves retraining the model using specific techniques such as reinforcement learning, curriculum learning, or adversarial training. Fine-tuning allows you to refine the model's behaviour and performance on specific tasks or domains.
Continuous Improvement: The process of training and fine-tuning ChatGPT is iterative. Collect user feedback on the model's performance in real-world scenarios and use it to improve it further. Consider implementing an efficient feedback loop to continuously update and refine the training data, prompts, or post-processing techniques based on user interactions.
Regular evaluation and fine-tuning are essential to ensure your trained ChatGPT model remains up-to-date, accurate, and aligned with your desired behaviour. The following section will provide some tips for efficient and quick ChatGPT training.
Tips for Efficient and Quick Training of ChatGPT
Training ChatGPT can be time-consuming, but you can employ several tips and techniques to make it more efficient and faster. Consider the following suggestions to streamline your training.
Hardware Acceleration: Use hardware acceleration, such as GPUs or TPUs, to speed up the training process. These specialized hardware devices are designed to perform matrix computations efficiently, which are fundamental to training deep learning models like ChatGPT. Hardware acceleration can significantly reduce the training time and make the process more efficient.
Batch Training: Train your model using mini-batches of data rather than individual examples. Batch training allows the model to process multiple examples simultaneously, leveraging parallelism and reducing the overall training time. Experiment with different batch sizes to find the optimal balance between training speed and memory requirements.
Early Stopping: Implement early stopping techniques during training to prevent overfitting and save training time. Early stopping involves monitoring the model's performance on a validation dataset during training and stopping the training process when the performance degrades. This helps avoid unnecessary training iterations and speeds up the overall process.
Transfer Learning: Leverage transfer learning techniques to accelerate the training process. Instead of training ChatGPT from scratch, start with a pre-trained model and fine-tune it using your specific dataset. Transfer learning allows the model to benefit from the knowledge learned on a large-scale dataset, reducing the training time while still achieving good performance.
By following these tips, you can optimize the training process and reduce the time required to train ChatGPT, making it more efficient and practical for real-world applications. In the next section, we will explore some tools and resources that can aid in training ChatGPT.
Tools and Resources for Training ChatGPT
Training ChatGPT requires access to suitable tools and resources. Fortunately, several libraries, frameworks, and pre-trained models are available to simplify and streamline the training process. Here are some notable tools and resources.
Hugging Face Transformers: The library provides a high-level API for training and fine-tuning transformer models, including ChatGPT. It offers various pre-trained models, tokenizers, and utilities that facilitate the training and deployment of ChatGPT.
OpenAI GPT-3: OpenAI GPT-3 is a state-of-the-art language model for training conversational AI systems. It provides an API that allows developers to interact with and train the model using custom prompts and datasets. GPT-3 offers powerful capabilities for natural language processing and generation.
Datasets: Several datasets are available for training conversational AI systems. The ParlAI framework provides access to various conversational datasets, including dialogue-based tasks and chat.
Conclusion
Training ChatGPT is a complex and multifaceted process that involves data collection, preprocessing, model architecture, training, and fine-tuning. The resulting language model offers powerful capabilities that can be leveraged in numerous practical applications, from customer support and content creation to virtual assistants and educational tools.
While AI language models are associated with challenges and limitations, ongoing research, development, ethical considerations, and bias mitigation efforts will help address these issues. The future of AI language models like ChatGPT is bright, with enhanced capabilities, integration with other technologies, and democratization efforts paving the way for exciting new possibilities.
By understanding how to train and utilize ChatGPT, individuals and organizations can harness the power of AI to drive innovation, improve efficiency, and create value across various domains. As we continue to explore the potential of AI language models, the possibilities are limited only by our imagination.